Gain new perspectives for faster progress directly to your inbox.
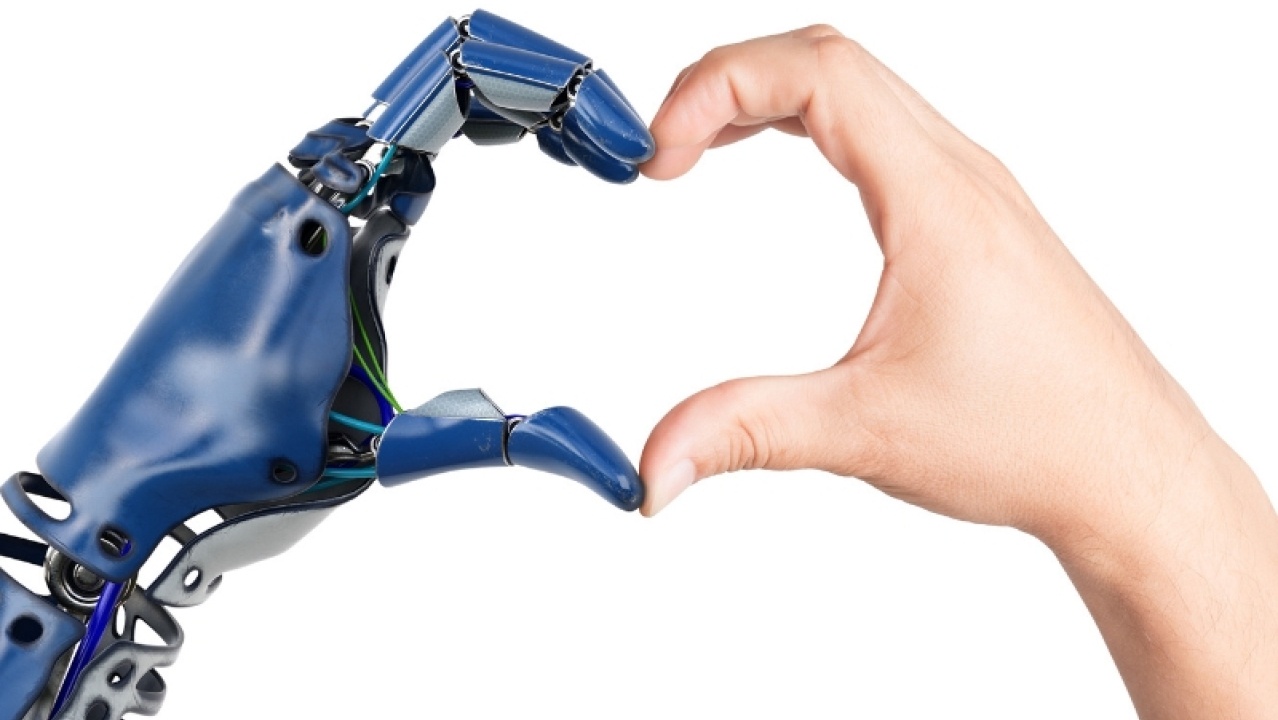
Artificial Intelligence: Big business
The artificial Intelligence (AI) industry is at the forefront of digital technologies and is transforming business to the extent that by 2023, it will have an estimated value of half a trillion USD. However, its advancement often raises a polarizing debate: That of man vs machine. Could robots push people out of jobs and take over our world?
According to an IDC report, worldwide spending in the AI market is set to grow 19.6% year-over-year in 2022 to $432.8 billion, leaving it on its way to breaching the $500 billion mark by 2023. IDC expects AI services to see the fastest spending growth at a compound annual growth rate (CAGR) of 22%, with AI platforms showing the biggest growth (34.6% CAGR) out of all AI software surveyed.
The AI drug development process
As standard, it now takes an average of 10–15 years for a drug to be identified, validated, developed and approved for clinical use. There are multiple challenges that come with bringing a new drug to market, the most notable being cost, which is estimated at $2.6bn with an approval rate of only 12%.
Drug discovery reinvented with AI
When it comes to digitalization, the pharmaceutical industry is at the forefront of cutting-edge research, capitalizing on the advancements that come with AI drug design. AI can recognize hit and lead compounds and can rapidly validate drug targets while also optimizing drug structure design.
Certain AI algorithms (e.g., Nearest-Neighbor classifiers, Radio Frequency (RF), extreme learning machines, Support-vector machines (SVMs), and deep neural networks) are used for virtual drug screening based on synthesis feasibility and can predict the physicochemical properties, bioactivity, and toxicity of target molecules, devoid of bias.
AI drug design can assist in structure-based discovery by predicting the 3D protein structure and providing vital information on the effect and safety of a compound prior to synthesis. AI methods have also been used to accurately predict ligand–protein interactions — ultimately ensuring better therapeutic efficacy. Prediction of drug–target interactions with AI has also been employed for drug repurposing and avoiding polypharmacology, which may relay substantial cost savings.
The involvement of AI in the de novo design of molecules can be beneficial due to its ability to provide online learning and simultaneous optimization of the already-learned data, as well as suggesting possible synthesis routes for compounds leading to swift lead design and development.
Finally, AI-enabled search solutions can play an important role across the entire patent ecosystem in improving both efficiency and patent quality. They can perform and review complex prior art searches, freeing time for patent examiners to undertake other tasks and reduce application delays.
Man and machine: Living in harmony?
Despite the myriad of applications in the AI drug development process, there are drawbacks to using AI in drug discovery, and human intervention will continue to be essential to the process. The quality of the predictions generated are largely dependent on the algorithm design. AI is also subject to algorithm bias, and algorithms must still be validated by scientists. While costs of supercomputing and high-throughput screening have decreased over time, they remain substantial.
One potential solution to such challenges is human-in-the-loop (HITL) AI, which combines the efficiency of AI and robotics with researchers’ input, ideas, and comprehensive judgement — saving time and resources while minimizing failures. This approach has been employed by Astellas, where one HITL method was proven to reduce the time from hit compound to acquisition of a drug candidate compound by approximately 70%. CAS has evaluated the structural novelty of the first three AI-designed drug candidates to enter human clinical trials. The first drug candidate, DSP-1181, was reported by Exscientia in early 2020. Two drug candidates — EXS21546 and DSP-0038 — have since followed suit with a pipeline of others rapidly progressing towards this milestone as several companies, including Exscientia, Insilico Medicine, and Schrodinger, are conducting preclinical, Investigational New Drug-enabling studies on potential candidates.
One cannot argue the benefits that AI drug development can bring to optimizing therapeutic discovery: AI can be harnessed to push the boundaries of technology when paired with innovative scientific thinking. Read more on this topic in our CAS Insights Report on the landscape of AI and chemistry.