Gain new perspectives for faster progress directly to your inbox.
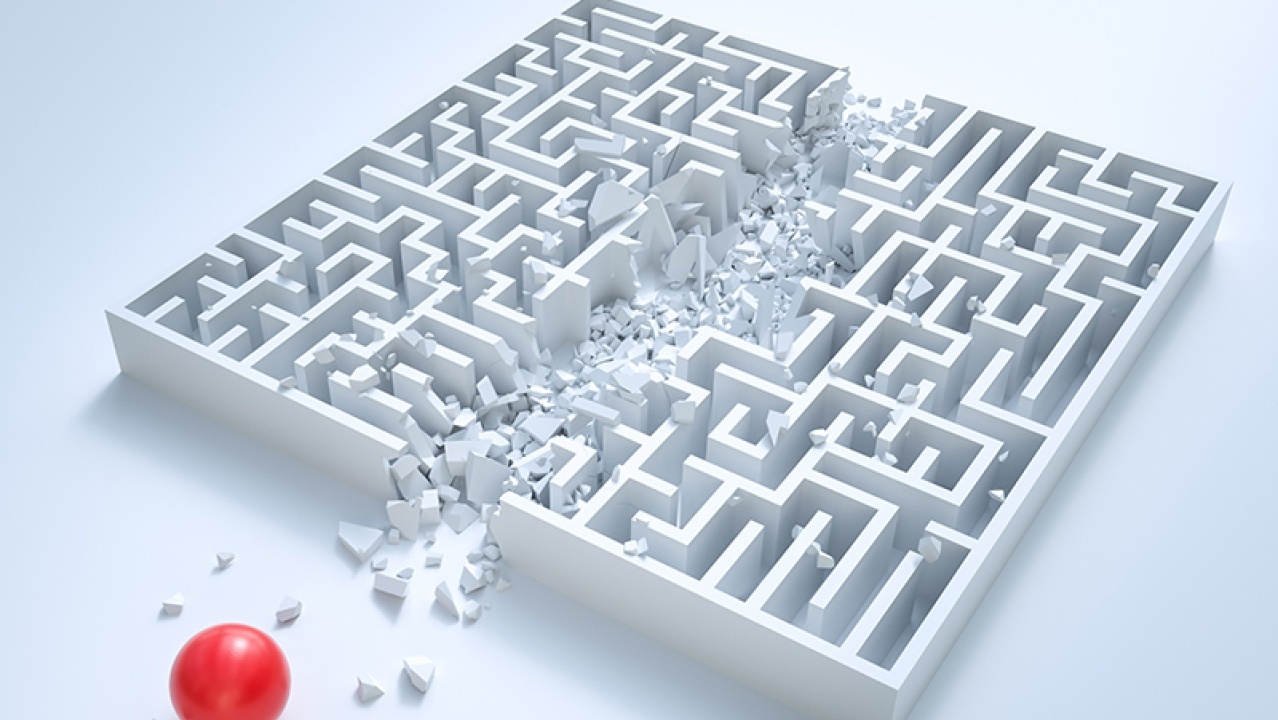
Created through the US FDA Safety and Innovation Act of 2012, Breakthrough Therapy Designation (BTD) was introduced to shorten the development and review time of promising new drugs intended to treat serious or life-threatening diseases for which there is an unmet medical need. The Breakthrough Therapy Designation approval pathway is distinct from other expedited development programs in that greater evidence of efficacy is required, but in return, sponsors receive much more substantive engagement and support from the FDA during clinical development. A key requirement for receiving Breakthrough Therapy Designation status is preliminary clinical evidence demonstrating substantial improvement on a clinically significant endpoint compared with other available therapies. Once designated as breakthrough therapies, investigational drugs receive intensive FDA guidance on an efficient drug development program, an organizational commitment to expedite the FDA development and review, and the potential eligibility, based on supporting clinical data, for rolling and priority review of the marketing application.
Receiving Breakthrough Therapy Designation status is considered a major accomplishment for any pharmaceutical R&D organization, bringing with it both public health and commercial benefits. Data shows that in addition to review periods being shortened, Breakthrough Therapy drugs will also spend a total of two to three years less in pre-market development compared with non-breakthrough therapy drugs. Furthermore, receiving this designation provides some credibility to the clinical promise of a given product and, as a result, can add significant value to a company. In fact, our analysis of publicly announced Breakthrough Therapy Designation grants found that the stock of publicly traded companies without any marketed products rose by an average of 6% (in excess of market returns) the day after Breakthrough Therapy Designation was announced.
The connection between chemical novelty and Breakthrough Therapy Designation
With such significant benefits, breakthrough therapy status remains a coveted but elusive prize. As of June 30, 2022, the FDAhad received 1265 total requests for Breakthrough Therapy Designation. However, only around 40% of these requests were granted.
The breakthrough status for a given drug is not disclosed by the FDA until it receives final approval. Between 2013 and 2019, just 73 (26%) of the 276 new therapeutic drugs (NTDs) approved by the FDA's Center for Drug Evaluation and Research (CDER) were granted BTD status. Small molecules were the dominant drug modality, representing 56% of these breakthrough NTDs. A majority of these FDA-designated breakthrough small molecule drugs included at least one structurally novel new molecular entity (NME) whose shape and scaffold were not used in any previously FDA-approved drugs. However, a closer look at the success rates for different types of small molecule drugs reveals some interesting findings.
Based on our recent analysis, approximately 3 in 10 structurally novel small molecule NTDs achieved breakthrough status compared with just 1 in 10 non-structurally novel small molecule NTDs. That means structurally novel drugs have been more than twice as likely to be granted Breakthrough Therapy designation status by the FDA. This gap highlights the differential impact of structural novelty and the importance of pushing the boundaries of chemical space even further in the search for new drugs.
In silico methods accelerating small-molecule drug innovation
Balancing innovation with efficiency is a longstanding challenge in drug discovery, as the pharmaceutical industry is under constant pressure to develop novel therapies that have significant clinical advantages over existing treatments. Structurally novel molecules have proven to be significantly more likely to be the source of promising new therapies. However, with the number of potentially synthesizable organic molecules estimated to be 10180 for those below 1000 Da, the exploration of the vast amount of chemical space in search of structurally novel drugs is beyond the reach of traditional experimental approaches.
Advancement of in silico methods is beginning to drive more efficient exploration of new biologically relevant regions of chemical space that contain structurally novel molecules. One of the primary in silico methods that many biopharma organizations have attempted to deploy over the last several years is machine learning. Machine learning can be used to predict the properties of a drug moleculewith an increasing degree of accuracy. This insight helps researchers prioritize the synthesis of drug-like molecules with more optimal property profiles and allows for the creation of more structurally diverse candidate pools of molecules representing larger portions of chemical space. These structurally diverse pools increase the odds of finding structurally novel drug-like molecules by providing a better selection of molecules that could be synthesized and assayed.
Advancing machine learning effectiveness in drug discovery
When it comes to building powerful predictive algorithms to explore chemical space broadly for drug discovery, the use of high-quality training data is key. Machine learning methods may draw on a range of different sources of public and internal, proprietary data. However, this disparate data must be refined, translated, structured, and indexed to unlock its true value. In fact, data scientists are still spending 38% of their time sourcing and cleaning up the data used to feed their algorithms – time that could be more productively spent developing models and optimizing results. Thus, the availability of data sets that are curated by human experts with experience in taxonomies, semantic linking, and data categorization can have a significant impact on the success of machine learning efforts in drug discovery.
Equally important are the molecular representations or ‘molecular fingerprints’ that are used to encode the structure of drug molecules in a form that is amenable to machine learning. Recent research showed that optimized fingerprints can make a significant difference in the accuracy of predictive models. Indeed, a new molecular fingerprint developed by data scientists at CAS improved prediction accuracy by up to 45% compared with the same algorithms running traditional Morgan fingerprint methods. These enhanced molecular fingerprints are already showing great promise in our drug discovery consulting projects to predict the biological activity of drug-like molecules, thereby helping to reduce the number of molecules that must be synthesized for screening and increase research efficiency in the search for the next generation of innovative therapeutics.
Interested in analyzing the structural novelty of your own intellectual property or optimizing your digital R&D initiatives? CAS Custom Services tailors our specialized technologies, scientific expertise, and unparalleled content to meet your unique needs.
How can CAS close knowledge gaps on emerging trends?
Stay on top of emerging approaches within drug discovery with CAS. Beyond structural novelty, small molecules are being used to find novel modes of targeted undruggable proteins. This has created new classes of therapeutic agents with exciting potential activity for many therapeutic areas. Learn more about the molecule glues drug discovery landscape, targeted protein degradation, and induced proximity in our latest white paper.