Gain new perspectives for faster progress directly to your inbox.
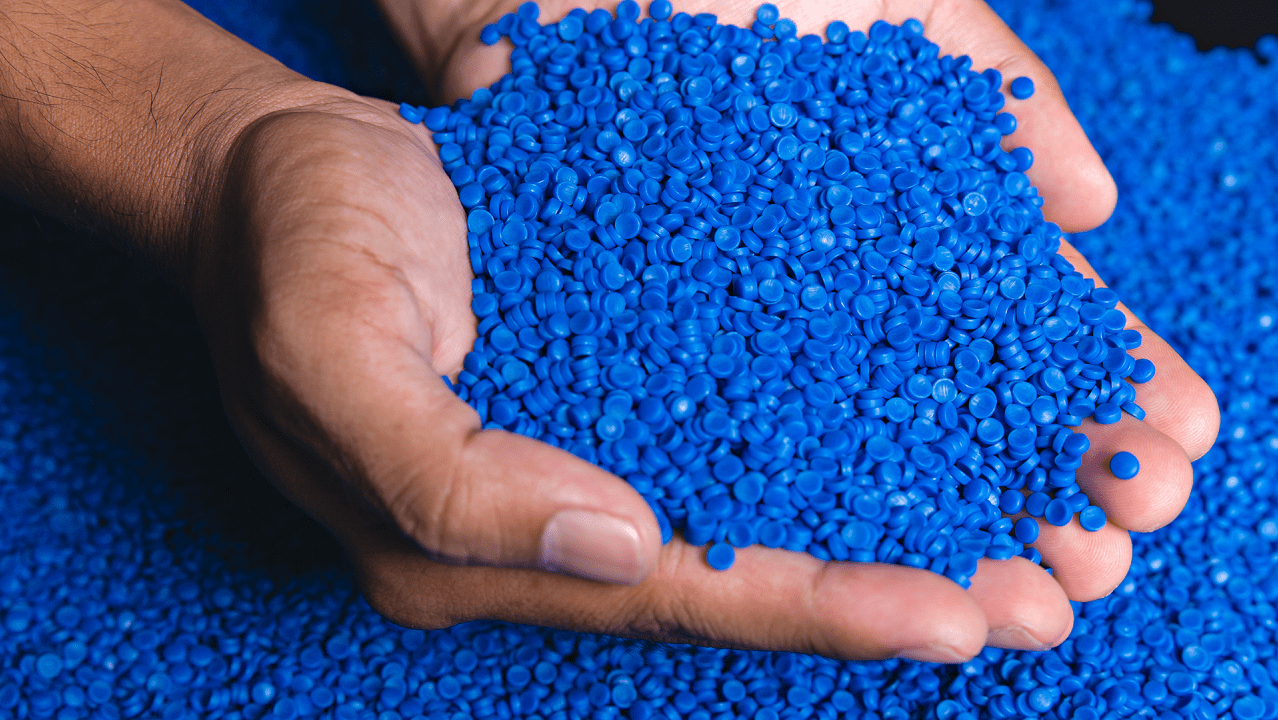
With almost 10% of the world’s fossil fuels going towards plastic production, a viable alternative to fossil fuel-based plastics has been a key goal for the past 20 years. Bio-based polymers, obtained from renewable biomass resources, have received wide attention as the ideal replacement. These polymers have been used to create bioplastics, which are a promising and sustainable alternative to oil-based plastics and could even benefit countries that are heavily reliant on foreign oil.
This journal manuscript in ChemRxiv details the three types of bio-based polymers, their strengths and weaknesses, the latest research progress, and the trends in this field of study. Because bioplastics often face skepticism from the public, likely attributed to misinformation, this article aims to clarify the confusions and raise the awareness of bio-based polymers’ importance to sustainability.